What is Edge AI: Benefits, How It Works & Applications
5/17/2024
What is Edge AI?
Edge AI refers to deploying artificial intelligence (AI) algorithms and models directly on edge devices, such as sensors, smartphones, or Internet of Things (IoT) devices, rather than relying on centralized cloud servers for processing. This enables real-time data analysis and decision-making at the edge of the network, without needing to transmit data to a remote server for processing. By running algorithms locally on edge devices, edge computing with artificial intelligence reduces latency, minimizes bandwidth usage, and enhances privacy and security, making it ideal for applications that require fast response times and efficient use of resources.
Why are We Moving to Edge AI?
The move towards Edge AI is fueled by the growing need for quick decision-making, automation, and efficiency across different sectors. Bringing AI to the edge allows for faster data processing, better data security, reduced network congestion, and the ability to work offline when internet connectivity is unavailable.
This shift is prompted by the increasing demand for automation, improvements in neural networks, advancements in computing, and the widespread use of IoT devices. Ultimately, AI and edge computing empower devices to make smarter decisions, leading to improved efficiency, safety, and overall effectiveness.
How is Edge Different from Other AIs?
Let's delve into the differences between Edge AI, Distributed AI, and Cloud AI, each offering unique ways to deploy artificial intelligence. We'll uncover their advantages, applications, and key considerations to help you better understand their distinct approaches.
Edge AI vs. Distributed AI
Edge AI enables devices to make decisions locally, reducing the need to constantly transfer data to a central location. However, for training and deploying AI models, data still needs to be sent to the cloud. Deploying edge AI across various locations faces challenges like managing large amounts of data and limited resources.
Distributed AI (DAI) addresses these challenges by collecting data intelligently and automating AI processes. DAI distributes tasks, coordinates performance, and scales applications across multiple systems and devices autonomously.
Unlike edge AI, DAI offers better scalability and flexibility, making it suitable for managing complex AI tasks across diverse environments.
Edge AI vs. Cloud AI
Edge AI and Cloud AI represent two different paradigms for artificial intelligence.
Edge AI involves processing data locally on edge devices, close to the data source. This method offers advantages like low latency, enhanced data privacy, and reduced dependence on network connectivity.
In contrast, Cloud AI involves centralizing the processing of data on remote cloud servers. While Cloud AI offers scalability, flexibility, and easy access to computational resources, it typically introduces higher latency and greater bandwidth usage due to data transmission over the internet. Additionally, Cloud AI may raise concerns regarding data privacy and security, as sensitive data must be transmitted and stored on external servers.
Edge AI |
Cloud AI |
|
Processing Location |
Local device |
Remote cloud servers |
Data Volume |
Limited |
Large |
Latency |
Low (real-time) |
High |
Power Consumption |
Low |
High |
Cost (Hardware/Software) |
Lower |
Higher |
Security |
Potentially lower |
Higher (centralized) |
Offline Functionality |
Yes |
No |
Ideal Applications |
Wearables, sensors, robotics, IIoT devices |
Facial recognition, big data processing |
In conclusion, Edge AI excels in real-time applications on local devices like wearables, IIoT devices, and security cameras, providing low latency and offline functionality. On the other hand, Cloud AI boasts powerful processing capabilities and vast data storage, making it ideal for complex tasks like facial recognition and big data analytics. By understanding the strengths and applications of each approach, it is possible to leverage the most suitable AI technology to meet specific needs and goals.
Benefits of Edge AI
Edge AI revolutionizes data processing on devices, offering numerous advantages:
Scalability
Edge AI offers great scalability, allowing organizations to easily expand their AI capabilities as their needs grow. Unlike traditional centralized AI systems, which may require significant upgrades to accommodate increased data volumes or processing demands, edge AI can scale horizontally by deploying extra edge devices. This enables smooth integration of new devices into existing infrastructure, ensuring efficient and cost-effective scalability without sacrificing performance or reliability.
Cost Effective
Edge AI reduces costs by processing data locally on devices, minimizing the need for expensive cloud infrastructure and reducing data transmission costs. This approach also eliminates the need for constant internet connectivity, further reducing operational network expenses.
Power Efficiency
Edge AI conserves power by conducting computations locally, minimizing data transmission over the network, and reducing reliance on energy-intensive cloud servers. Additionally, edge devices are designed to be more energy-efficient and sustainable compared to their cloud counterparts. This approach also leads to an improved carbon footprint for edge devices by reducing the number of I/O operations required by cloud AI applications.
Enhanced Data Security
Edge AI enhances data security by processing sensitive information locally on devices, reducing the risk of data exposure or attacks during transmission to cloud servers. This approach ensures that critical data remains within the confines of the device, mitigating the threat of unauthorized access, data breach, or interception.
Less Bandwidth
Edge AI minimizes bandwidth usage by processing data locally on devices, reducing the volume of data that needs to be transmitted to the cloud for analysis. This results in optimized network bandwidth utilization, lower data transfer costs, and reduced network congestion, particularly in environments with limited bandwidth availability.
Reduced Latency
Edge AI also significantly reduces latency by processing data locally on devices. Just like the bandwidth, it eliminates the need to send data to remote cloud servers. This enables real-time decision-making and responsiveness, making Edge AI ideal for applications requiring immediate action, such as autonomous vehicles and industrial automation.
Real-time Performance
Edge AI enables high-performance computing directly on local devices, processing data, conducting instantaneous analysis, and running Machine Learning and Deep Learning algorithms. Unlike cloud processing, edge data processing takes milliseconds, making it ideal for critical real-time applications like defect detection in production lines and abnormal behavior detection in security systems.
On-Device Learning
Edge AI supports on-device learning, allowing devices, like microcontrollers, to continuously improve their performance and adapt to changing environments without requiring constant access to cloud-based resources. This enhances the intelligence and autonomy of edge devices by enabling autonomous operations and personalized user experiences.
Improved Privacy
Edge AI operations conduct most data processing locally, minimizing data sent to external locations or remote cloud servers, reducing the risk of data mishandling. This enhances privacy, vital for sensitive data like security, IP, and other private information.
High Availability for Devices
Edge AI ensures high availability for devices by enabling them to operate autonomously without relying on continuous internet connectivity or cloud-based services. This means that even in remote or disconnected environments where network connectivity may be limited or unreliable, edge-based applications can continue to function effectively. Reducing dependency on external resources, Edge AI can ensure uninterrupted functionality and responsiveness, vital for mission-critical applications.
How Does Edge AI Technology Work?
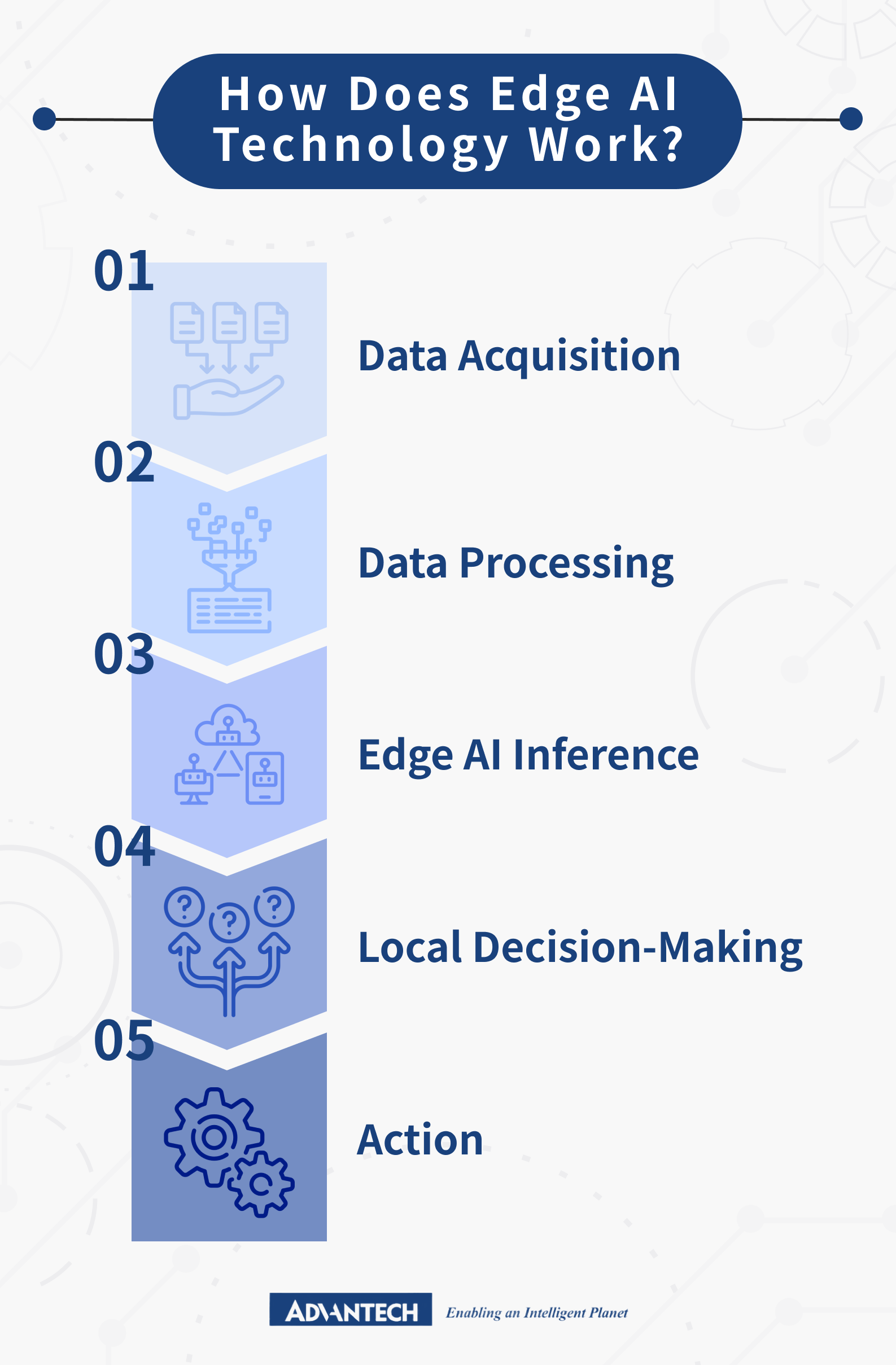
Edge AI devices follow a systematic process to analyze data and make real-time decisions at the edge of the network. Let’s take a look:
- Data Acquisition: Sensor devices capture raw data from the surrounding environment.
- Data Preprocessing: Raw data is preprocessed locally to filter noise and extract relevant features.
- Edge AI Inference: Preprocessed data is fed into the Edge AI model deployed on the edge device.
- Local Decision-Making: The Edge AI model analyzes the data and makes real-time decisions or predictions locally.
- Action: Based on the inference results, immediate actions or responses are triggered, enhancing efficiency and responsiveness.
In essence, Edge AI technology brings intelligence closer to the source of data, unlocking new possibilities for real-time operation and automation in all industries.
Examples of Edge AI
Manufacturing Sector
Edge AI transforms manufacturing with predictive maintenance and process optimization in smart factories. AI-equipped edge devices analyze sensor data to detect equipment failures and defects in real time, enhancing productivity and minimizing downtime. This proactive approach to maintenance, particularly in IIoT devices, allows for early anomaly detection and predictive analysis, ensuring efficient production processes and cost savings through timely repairs.
Healthcare Industry
In healthcare, edge AI enhances patient care by enabling real-time monitoring, diagnosis, and personalized treatment. Wearable devices, like cardiac trackers and blood pressure sensors, embedded with AI algorithms continuously monitor vital signs and analyze data locally, allowing for immediate detection of health issues and timely intervention. Also, with machine learning, AI systems swiftly and accurately examine medical images. This capability assists in spotting diseases at early stages, allowing for timely interventions that can potentially save lives and enhance treatment effectiveness.
Smart City Solutions
With Edge AI, smart cities can achieve efficient infrastructure management, traffic optimization, and public safety. Embedded within urban infrastructure like traffic lights and surveillance cameras, edge devices analyze data locally to identify traffic congestion, monitor environmental conditions, and track suspicious objects for enhanced security. This localized processing enables cities to improve public services, enhance safety measures, and allocate resources effectively, ultimately fostering a more sustainable and livable urban environment.
Smart Home Technology
Smart home devices with edge AI capabilities, like voice-controlled appliances and video doorbells, process data locally to recognize patterns and personalize user experiences without cloud reliance. For example, a smart fridge tracks food consumption and expiry, offering timely alerts. These smart home devices boost privacy, security, and overall living quality.
Retail Industry
In retail, edge AI drives customer engagement and enhances inventory management through real-time analysis of shopper behavior. Smart shelves with edge AI sensors optimize product placement and offer personalized promotions, improving operational efficiency and reducing costs. Additionally, voice ordering enhances the digital shopping experience, allowing shoppers to search for items, request product information, and place orders using smart devices.
Energy Sector
In the energy sector, Edge AI optimizes distribution, predicts demand, and enhances grid efficiency by combining historical data, weather patterns, and energy health data. Smart grids use AI-equipped edge devices to analyze real-time data, detect anomalies, and optimize energy flow, reducing waste and improving reliability. This ensures cost savings and sustainable infrastructure, which is critical for continuous energy supply and efficient resource management.
Future of Edge AI
As we gaze into the future of Edge AI, a realm of limitless possibilities unfolds. With advancing technology, Edge AI algorithms are poised to tackle complex tasks with remarkable efficiency. The convergence of factors like neural network maturity, IoT proliferation, and 5G technology leads us into a new era, setting the stage for enterprises in every field to seamlessly integrate AI and use real-time insights to enhance operations across sectors. The future of Edge AI holds incredible promise, bringing innovations that will reshape industries and fuel unprecedented growth.
To explore the exciting possibilities of Edge AI and witness its real-world applications in action, check out Advantech’s remarkable success stories in deploying Edge AI solutions. Experience the transformative power of Edge AI and unlock new opportunities for your business. Step into the future today with Advantech!